Increasingly these days drones are being used for urban surveillance, delivery, and examining architectural structures. To do this autonomously often involves using “map-localize-plan” techniques wherein first, the location is determined on a map using GPS, and then based on that, control commands are produced.
A neural network that does steering and collision prediction can compliment the map-localize-plan techniques. However, the neural network needs to be trained using video taken from actual flying drones. But generating that training video involves many hours of flying drones at street level putting vehicles and pedestrians at risk. To train their DroNet, Researchers from the University of Zurich and the Universidad Politecnica de Madrid have come up with safer sources for that video, video recorded from driving cars and bicycles.
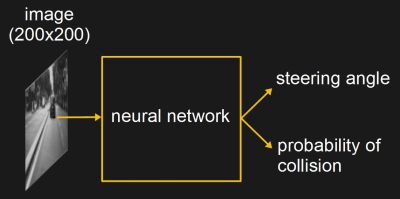
For the drone steering predictions, they used over 70,000 images and corresponding steering angles from the publically available car driving data from Udacity’s Open Source Self-Driving project. For the collision predictions, they mounted a GoPro camera to the handlebars of a bicycle and drove around a city. Video recording began when the bicycle was distant from an object and stopped when very close to the object. In total, they collected 32,000 images.
To use the trained network, images from the drone’s forward-facing camera were fed into the network and the output was a steering angle and a probability of collision, which was turned into a velocity. The drone remained at a constant height above ground, though it did work well from 1.5 meters to 5 meters up. It successfully navigated road lanes and avoided moving pedestrians and bicycles. Intersections did confuse it though, likely due to the open spaces messing with the collision predictions. But we think that shouldn’t be a problem when paired with map-localize-plan techniques as a direction to move through the intersection would be chosen for it using the location on the map.
As you can see in the video below, it not only does a decent job of flying down lanes but it also flies well in a parking garage and a hallway, even though it wasn’t trained for either of these.
How close are we to having delivery drones? Medical deliveries by drone are already happening in Rwanda. but mostly we’ve seen experiments and demonstrations for safe handling by the customer or keeping the drone out of the customer’s hands altogether by dropping the parcel using a parachute.
Why, rather than being trained by vehicles, can the drones not instead take inspiration from nature and just fly OVER things?
Flying over things is easy. Flying around and between things is hard, which what this training is for.
Perhaps wind? Large cities produce quite an updraft around skyscrapers, this may be more than a drone can handle without appreciable loss of flight time or risk losing the payload. There’s also NOTAMS and restricted air space associated with hospitals and other helipads you may have to fly around at different times of the day/year.
Now I want to attach a thermal camera to a glider in some city-like textured place (canyon?) and detect updrafts from light reflected off of flat surfaces.
Future’s going to be interesting indeed. Soon, instead of the soothing sounds of nature, the sky’s will be filled with the soothing sounds of drones. Lots and lots of drones.
“Lots and lots of drones.”
…attacking each other as companies compete for delivery contracts.
At least we’ll have lots of free parts!
You mean “Skeet”.
“A neural network that does steering and collision prediction can compliment the map-localize-plan techniques.”
“Hey, that’s some sweet map-localize-plan technique you’ve got there!”
Long ago I saw an item how Google streetview actually had bikes with cameras for places cars can’t go, then later they even mentioned a backpack device.
All cute and well but years later when I check streetview I only see view of roads where cars can go and perhaps a dozen specially picked tourist spots worldwide where they got off the motor vehicle roads.