AI agents are learning to do all kinds of interesting jobs, even the creative ones that we quite prefer handling ourselves. Nevertheless, technology marches on. Working in this area is YouTuber [AI Warehouse], who has been teaching an AI to walk in a simulated environment.
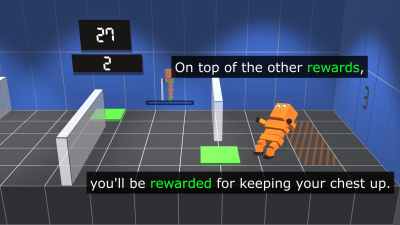
The AI controls a vaguely humanoid-like creature, albeit with a heavily-simplified body and limbs. It “lives” in a 3D environment created in the Unity engine, which provides the necessary physics engine for the work. Meanwhile, the ML-Agents package is used to provide the brain for Albert, the AI charged with learning to walk.
The video steps through a variety of “deep reinforcement learning” tasks. In these, the AI is rewarded for completing goals which are designed to teach it how to walk. Albert is given control of his limbs, and simply charged with reaching a button some distance away on the floor. After many trials, he learns to do the worm, and achieves his goal.
Getting Albert to walk upright took altogether more training. Lumpy ground and walls in between him and his goal were used to up the challenge, as well as encouragements to alternate his use of each foot and to maintain an upright attitude. Over time, he was able to progress through skipping and to something approximating a proper walk cycle.
One may argue that the teaching method required a lot of specific guidance, but it’s still a neat feat to achieve nonetheless. It’s altogether more complex than learning to play Trackmania, we’d say, and that was impressive enough in itself. Video after the break.
Now, teach Albert how to launch nuclear missiles.
Skynet is waiting…
C’mon, attach AI to QWOP. I’d watch that for a couple hours.
Attach him to WOPR.
Code Bullet did something similar. Here’s part one, https://youtu.be/qvpXpCvkqbc
I wonder, if the “guidances” were given either directly (withou first learning to worm and skip) or not at all (probably: always worming) whether albert wouldn’t have reached his goal faster. Evolution works random like that.
What is my purpose?
…to make paper clips.
Oh. My. God.
@AI Warehouse said: “Decaying plasticity was a big issue. Basically, Albert’s brain specializes a lot from training in one room, then training in the next room on top of that brain is difficult because he first needs to unlearn that specialization from the first room. The best way to solve the issue is by resetting a random neuron every once in a while so over time he “forgets” the specialization of the network without it ever being noticeable, the problem is I don’t know how to do that through ML-Agents. My solution was to keep training on top of the same brain, but if Albert’s movement doesn’t converge as needed I record another attempt trained from scratch, then stitch the videos together when their movements are similar. If you know how to reset a single neuron in ML-Agents please let me know! The outcome from both methods is exactly the same, but it would be a smoother experience having the neurons reset over time instead of all at once.”
This is the problem with ML as it is today; once it learns something wrong, aside from a global reset, it’s next to impossible to unlearn the wrong behavior. Eventually, the corpus of what good has been learned has taken so much time (and time is money), global resets become too expensive to consider. In this case the next best option is to selectively attempt to train-over the bad behavior until it goes away (a long therefore expensive process). But in truth, it never really goes away!
It’s like choosing what side of the road you drive on, or your electricity supply voltage and frequency and plug. Sunk costs in simulation matches sunk cost in real life.
So what I’m seeing is A.I. is basically a million monkeys at a million typewriters, just a heck of a lot faster.
Also, don’t be surprised when a terminator made of paper clips says “this one’s for Albert” right before blowing you away.
Don’t anthropomorphise the robots. They don’t like it.
LOL!