One of the persistent challenges in audio technology has been distinguishing individual voices in a room full of chatter. In virtual meeting settings, the moderator can simply hit the mute button to focus on a single speaker. When there’s multiple people making noise in the same room, though, there’s no easy way to isolate a desired voice from the rest. But what if we ‘mute’ out these other boisterous talkers with technology?
Enter the University of Washington’s research team, who have developed a groundbreaking method to address this very challenge. Their innovation? A smart speaker equipped with self-deploying microphones that can zone in on individual speech patterns and locations, thanks to some clever algorithms.
Robotic ‘Acoustic Swarms’
The system of microphones is reminiscent of a swarm of pint-sized Roombas, which spring into action by deploying to specific zones in a room. Picture this: during a board meeting, instead of the usual central microphone setup, these roving mics would take its place, enhancing the control over the room’s audio dynamics. This robotic “acoustic swarm” can not only differentiate voices and their precise locations in a room, but it achieves this monumental task purely based on sound, ditching the need for cameras or visual cues. The microphones, each roughly an inch in diameter, are designed to roll back to their charging station after usage, making the system easily transportable between different environments.
The prototype comprises of seven miniature robots, functioning autonomously and in sync. Using high-frequency sound, much like bats, these robots navigate their way around tables, avoiding dropoffs and positioning themselves to ensure maximum audio accuracy. The goal is to maintain a significant distance between each individual robotic unit. This spacing increases the system’s ability to mute and create specific audio zones effectively. The sound from an individual speaker will reach each microphone at different times. Thus, the greater distance between microphones makes it easier to triangulate that person’s location and filter them out from the pack. Regular smart speakers often have arrays of many microphones, but as they are separated by only a few inches at most, they generally can’t achieve the same feat.
“If I have one microphone a foot away from me, and another microphone two feet away, my voice will arrive at the microphone that’s a foot away first. If someone else is closer to the microphone that’s two feet away, their voice will arrive there first,” explained by paper co-author Tuochao Chen. “We developed neural networks that use these time-delayed signals to separate what each person is saying and track their positions in a space. So you can have four people having two conversations and isolate any of the four voices and locate each of the voices in a room,” said Chen.
Tested across kitchens, offices, and living rooms, the system is capable of differentiating voices situated within 1.6 feet of each other 90% of the time, without any prior information about how many speakers are in the room. Currently, it takes roughly 1.82 seconds to process 3 seconds of audio. This delay is fine for livestreaming, but the additional processing time makes it undesirable for use on live calls at this stage.
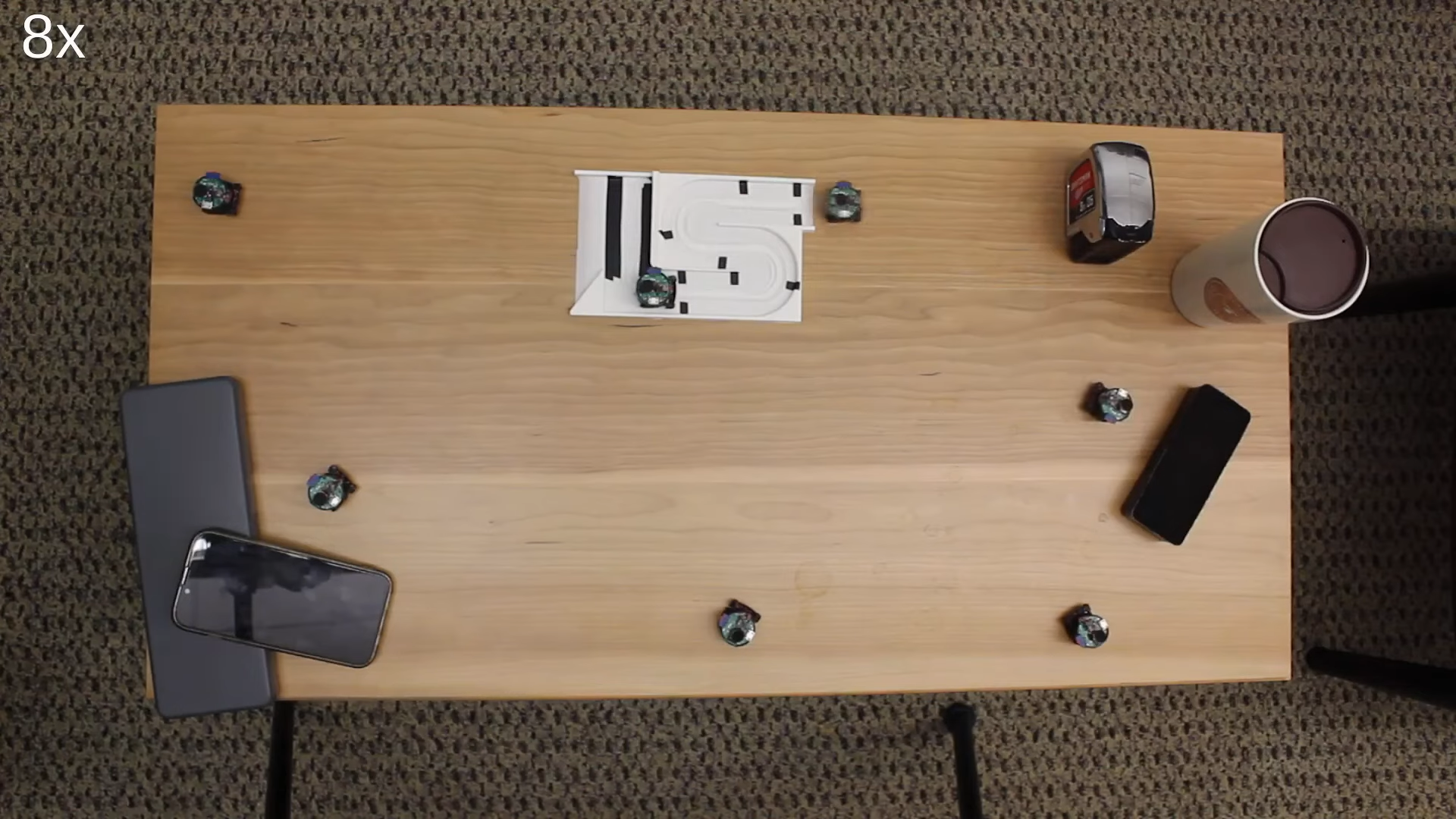
The technology promises to have great application in a variety of fields. A smart homes equipped with a well-spread microphone array could permit vocal commands solely from individuals in designated “active zones.” A voice-activated TV could be set up to only respond to instructions from those seated on the couch. It could even allow a group to take part in a virtual conference from a noisy cafe without everyone having to put on a microphone. It’s an edge case, sure, and you’d still need headphones, but someone’s likely to try it, right?
The team hopes to further develop the concept with more capable microphone ‘bots that can move around a room, not just a table. The team is also exploring using the robots to emit sounds to create “mute” and “active” zones in the real world, which would allow people in different parts of the same room to hear their own audio feed.
While the technology is still in an early research stage, we kind of love the idea. Many a corporate meeting could be livened up with a few cute robots skittering around, even if it’s ostensibly for the purpose of capturing better audio.
No thanks. Creepy and distracting. I’d rather have little robots like this top off my coffee during a meeting.
What if each one had a biscuit on top?
The problem is not unlike the one confronted by hearing aid users, signal detection and noise suppression. Many of he guys in my cohort (80++) are getting them and many are quite pricey and purport to have ai capabilities. Some of them can be managed from cell-phone apps.
I would think that having a “waterfall display” like some signal analyzers provide but instead displaying the range of “organized” and hopefully characterized sounds within range such that what looks like a notch filter, or several noth filters could remove that which is the noise du jour.
a frequency waterfall would have been suifficient to notch out the very annoying woman sitting at the next table. The discussion underway included recognizing this possibility whereupon freind’s wife pointed out that every adult male known to her was able to filter out women’s voices without any fancy expensive equipment.
I still think this would be an opportunity for applying deep learning to the development of a display where different noises can be displayed ver the range of audible sound and the unwanted ones notched out.
If I was a lot younger, I think I’d try to do this.
You shouldn’t need to manually twiddle with the frequency response of your hearing aids. They should automatically filter out constant noises (ought to, it can be relatively easily done.)
I watched my brother-in-law fiddling with his hearing aid settings through his phone recently – it looked terribly inconvenient.
My brother-in-law is a nice guy and knows a lot about insurance. He knows jack about technology. I really can’t imagine teaching him how to read a waterfall diagram and adjust his hearing aids from it.
Somebody ought to make a hearing aid with a beam forming rig in it. It should point straight ahead – turn your head and look at someone to focus on what that person is saying.
It ought to be possible to make a hearing aid that doesn’t require a phone and PhD to operate.
HI Joseph,
imagine looking at an ai driven waterfall. there will be vertical streaks which run from top to bottom. they will start and stop with the sound of interest. and if it someone talking, you land your finger on the track and the software will mute it. maybe the track will turn color so you can touch it again if you decide to hear it. the horizontal axis of the waterfall moight be something other than frequency, but the vertical could be loudness or solmething else.
the ai would characterize the various sounds into a form which could be displayed as a vertical track on the waterfall. Somehow it doesn’t seem to be that much of a stretch to suppose that ai could reduce a particular voice or noise to a single track on a waterfall.
Um, Ok. I wear hearing aids and work in IT infrastructure for more than 30 years. I’ll be happy to help you out, whether in designing them, testing them, or just buying them. I’ve never worked in that specific space before though.
It’s not so easy though. Even lately with reduced regulations, there’s a lot to take into consideration to sell a product on the medical market. At least now, you can make something and sell it around the medical market without all those approvals, but hey, it still has to work unless you’re a crook, in which case I won’t help you.
HI Tom,
Not me. I don’t have time. I’m up to my ears in autonomous fixed wing aircraft which at least do things I can see.
Having watched a friend try to mute a couple of very specific noise sources which were preventing a conversation we were attempting as he fussed with what was a $6,500 +/- hearing aid which supposedly could do it all itself but wasn’t cutting it, it seemed to me an AI project which via resident deep learning setup would listen to the noise-stream identified by touching the screen, maybe change color when system felt it had a good handle on that stream and then mute it with an addtional touch. This isn’t simply a frequency problem but I think a problem well within the state of the art for such things.
Maybe somone is doing this now?
I agree, john, it’s going to take deep learning to solve it. My hearing aids are top of the line, but the audio steering and noise suppression really isn’t that useful. As much as they are big advances over my grandfather’s battery-eating, sometimes whistling, always too loud or too soft hearing aids. there is still plenty of room to innovate. To get there we will probably need a few more sensor inputs than the 4 or 6 microphones that fit on two hearing aids. Now combine that with the phone in your pocket, and the watch on your wrist, and ideally some video source such as cameras mounted on eyeglasses, and it starts to get interesting.
If anybody is working on that commercially, they aren’t advertising to me, and I am definitely their target audience.
So basically a complicated way to say they implemented beam forming. The microphones don’t need to move around, you just need an array and you can record everyone and selectively pick every conversation digitally, the same way you can do that with RF. You could potentially isolate every speaker at the same time and even track their movement within the room. Using robots that move around seems overcomplicated, but a lot more fun to do for a group of students.
I feel like I’m missing something:
What’s the scenario in which a microphone on a robot for each speaker is preferable to a lapel microphone?
It’s not “a microphone on a robot for each speaker.”
It’s “swarm of mobile bots position themselves to better perform beam forming to target a specific speaker.”
I don’t know when you’d need that, either.
It looks like a solution searching for a problem.
On the other hand, the researchers got some experience with beam forming and robots – that’s got to count for something.
“It looks like a solution searching for a problem”
Agreed. The solution is to simply wait your turn to speak. Although sometimes that is hard to do.
I don’t see how this concept wins over having a static microphone array for the conference table – beam steering from that array to all the seats in the room for instance is pretty trivial computationally but will filter out directionally very well. So can split a room of noise into x separate microphone spots in effect, add a little volume levelling for each position if you like, again not that computationally intensive. Best of all no little robots to distract when they suddenly decide it is time to move or end up in the way of putting down your cuppa! As all you need is a relatively small array that can be fixed in the middle of the table, on a wall, celing etc.
The fixed array seems both simpler and at least as effective as the bot approach. A ceiling mounted or suspended array would probably be better. Table mics tend to pick up a lot of paper shuffling noise.
I think a lot of the comments above about beamforming are neglecting a few challenges with audio that make this more than a beamforming problem.
TLDR: It’s not that simple. The ear-brain combination is pretty amazing at its peak. It takes a neural network to really do this stuff right.
I have studied a fair amount about this stuff both in academics (EE signal processing focus) and in my professional career (many years working with undersea sonar). Ever since I took my first DSP class, I have wanted to make a beamforming active noise cancellation “cone of silence” composed of a ridiculous number of small audio transducers mounted on the walls and ceilings in restaurants, airports, etc., that could cancel out all exterior sound for each person except within their desired cone of hearing… so talk to the person next to you, but don’t even hear the next table over. As I gained experience on the subject I grew less and less hopeful of ever getting it to work.
The fundamental components of human speech are reportedly at frequencies of 85 Hz to 255 Hz, while voiced harmonics and non-voiced sounds can reach 4 kHz and higher. Thus the wavelengths range from 4 meters down to 8 cm or less. The rule of thumb for simple beamforming is that your 3dB beamwidth is 50 * wavelength / array length. So to get a beamwidth of 30 degrees, which is like your phone camera at 2x zoom, at the longest wavelengths you need a 6.7 meter array of microphones/speakers. But you still have sidelobes only 13dB down, so to suppress those further you need a weighted window function, aka shading. But shading increases mainlobe beamwidth. How much suppression do you need? The undamaged human ear has pretty good dynamic range, around 120 dB. To fully mute non-desired sounds such as the person at the next table, we need to suppress the intensity of undesired sound below the ear’s frequency-dependent threshold of sensitivity, which fortunately for the cone of silence is up to 50 dB higher (less sensitivity) at the lowest speech frequencies than it is at the ear’s peak performance frequency (~2kHz). This is maybe 10dB below normal talking volume, so it doesn’t take much to suppress extraneous sounds at the lowest frequency… may not need the full 6.7 meter array. Unfortunately the ear is very sensitive at the middle and higher speech frequencies. At 300Hz you need to suppress at least 30dB, and that still requires a big shaded array. Of course I am ignoring a LOT of stuff here… need to look at spherical spreading, reflections, multipath through body or other solid objects, SINR, etc.
So how in the world do our 2 little ears do so well when the math says we need an array of 3 meters or more? The brain does a lot more audio processing than just beamforming, and gets a tremendous advantage from the opposing separated ears. This goes beyond my professional expertise, but I happen to have firsthand experience with it because I lost all hearing in one ear a few years ago. I wear a snazzy bi-cros hearing aid setup… audio from the deaf side is routed to the hearing side, and mixed with amplified local sound. Now that I have only single-channel hearing I find source separation / suppression is virtually impossible. If two people are talking in a room, unless I can get my good ear within arms length of their mouth, I can’t figure out a word that is said. It’s called the cocktail party problem in the literature, and as bad as it was for me before the deaf ear, its comically bad now.
The good news for the cone of silence and the table-crawling microphones above is that there are more ways to try to solve it than just frequency filtering or beamforming. The best algorithms a decade or two ago were variants of independent component analysis (ICA), which is statistical in nature… try to minimize the correlation between various weighted and delayed sums of the inputs. These days, like the UAV enthusiast in the comments above said, all the kids are using deep learning approaches, and getting impressive results. Maybe when I retire I will get that cone of silence working after all. Or I will just turn off my hearing aids and enjoy the ham radio in my head… the tinnitus in my deaf ear is a veritable auroral symphony.
A solution emerged from the covid crisis, with home teleconference. The mic-headphones helped a lot to provide easier speech listening.
I remember headaches with 90’s conference phones, random voices and crappy speakers.
In the meering rooms, many attendees use now a headset connected to the laptop. Surprisingly, the listening comfort is better at home than in the conference room. A cheap electret and the appropriate position provide a sound near broadcast grade. As hearing impared worker, I improved the meetings with high end heaphones. I selected a model with no larsen on the hearing aids.
I have hearing loss. I built a hearing aid using high quality headphones with microphones amps and simple filtering that works great cost under $100. Voices sound mostly like I remember them and most music is ok. I have high end hearing aids that can connect to bt for phone calls and have digital frequency response calibration cost direct from China under $100. The problem of cocktail party conversations could be solved with AI but not until we can explain what we want AI to do.
With the caveat that what follows is naive, It seems to me that “hearing aid” systems are susceptible to a number of recent innovations, including in addtion to the “ear piece” a microphone on a wristwatch in addition to the one on the cell phone which might be carried in a shirt pocket and each of whose signals could be processed in the hope of isolating signal from noise.
To return to my scheme for rejecting noise by picking out its AI characterized track on a watefall display on the cell phone, maybe there is a better scheme. This would involve choosing the desired signal or signals themnselves which the AI Tensor-Flow program would then characterize and generate algorithm(s) which would reject – or significantly reduce – everything else.
You would choose what you want to hear, instead of the machine trying to guess what you don’t want to hear.
I expect that voice is where the problem is most vexing and suppose that the algorithms used to identify individual speakers from telephone recordings might include elements which could be used in a Deep Learning routine.
Does this make any sense?
Yes, I think you have the gist of it. Take a look at the recent ads for Google’s “audio eraser” on the Pixel 8. There are undoubtedly other similar solutions, but the new ads show the idea… the deep learning model identifies various sounds, and the user selects the one they want to erase. I need to find a suitable model and try it out myself.
I’m amazed at having independently guessed how it should be done although I’d still go with picking what I wanted to hear. Narg, is voice intereference a bigger problem than “background noise” ie. having two or more “signals” one or more of which must be suppressed.
I don’t know for sure, but my intuition is that it is more challenging to isolate a single human voice mixed with several other competing human voices than it is to isolate a voice from different types of noise. Many of the signal characteristics will match or overlap for human voices, so the separation technique will have to be nuanced. I spent a little time asking ChatGPT about it and he said the same thing, which is wonderful confirmation bias, haha.
Interestingly, my ability to separate well-known voices such as family from competing voices has degraded severely with my single-side hearing. I would have thought I would still be able to pick my wife or kids voices out of a crowd, but my experience has been that the brain is really hindered once it only has a single audio stream to work with. I wonder if it would have been different if I lost the opposite ear, and hence a different brain hemisphere would have been involved.
Hi Narg,
I suspect a hindrance to the development of Deep Learning algorythms directed to voice identification is probable lack of library ot tens of thousands of voices, like Google’s library ot tens of thousands of flowers, dogs and cats. Despite my shaky credentials for the course I took a summer camp in Deep Learning at Florida Atlantic University. It was excellent. We were given problems to become familiar with TensorFlow. It helped to be sharp on Python.
The protocols for getting anywhere with this sort of thing range from knowing what you are looking for to having no idea in which case you are looking for Deep Learning to discover the characteristics of the members of the library that you might sort them on criteria you hadn’t expected, or known about.
The question then, is how can you distinguish different voices using lousy audio? If that can be systematized, you are in a better position to promote the voice/voices you are interested in and mute the rest of them.
I have a couple of questions.
If the processed voices you hear in the system you are now using are not too good, would it make more sense for the machine to synthesize voices that would really work for you? This would be instead of trying to clean up the audio input and transform it into something you can work with.
Do you know how you came to lose hearing in onse ear. At 81, my left ear is not as good as the right, which I ascribe to years of driving VW’s with the left window open.
And there were the airplans, but not so much because I cought on to headsets early. “What’s that you say/” “You used to fly Beeech 18’s??” “YOU USED TO FLY BEECH 18S?”
It also would be interesting to see if different age groups have different voice, or inflection patterns, as in the 20 and 30 year old cohort women who frequently end every statement with an upward inflection. It would also be interesting to see to what degree family members voice alike.
This is in response to your most recent comment, John, but replying to previous since we apparently hit the nested-reply limit.
Yes, for me at least, a synthesized voice providing the same information would be very welcome if the original voice’s audio was too poor quality. This could take advantage of other information such as visual lipreading, which apparently works reasonably well (search “papers with code lipreading”). It would need to be very low latency for real-time conversation. A “replay” function could also be useful, such as when you can’t ask the person to repeat themselves. Live closed-captioning in AR glasses could serve both purposes.
My hearing loss was post-surgical. I had a benign inner ear tumor called a vestibular schwannoma, which was causing 10dB or more of hearing loss on that side vs the other ear, along with diplacusis and sporadic vertigo. I elected for surgical removal in an attempt to save hearing and facial nerve function. The removal was successful, but the surgeons were unable to save the hearing nerve as the tumor had subsumed it.
Glad you were able to take that class, sounds really useful.
Hi Narg,
The Deep Learning examples I did for the course all involved processing images a few pixels at a time. I don’t know if this is the only method for sorting instances, and the deep learning really was sorting more than anything else.
Clearly audio signals can be readily converted to images and that may be the route that the invention of more intelligent hearing devices will need to take.
I really don’t know enough to generate any better ideas. Good luck with your situation.
john
We who live in the twenty first century and speak Metric would like to invite you.
this is actual still a problem of hearing aids, the not really able to filter the voice of somebody to then transfer it to a another frequency.
This is blind source separation and in theory having a microphone array that is adaptive can make the separation easier and thus faster computationally. Really fascinating problem but like other people have said it’s a solution in search of a problem. Honestly this would be better for surveillance applications like deploy them in a room during with a group of targets to follow all the different conversations happening and the locations of everyone in the room.