Segment Anything, recently released by Facebook Research, does something that most people who have dabbled in computer vision have found daunting: reliably figure out which pixels in an image belong to an object. Making that easier is the goal of the Segment Anything Model (SAM), just released under the Apache 2.0 license.
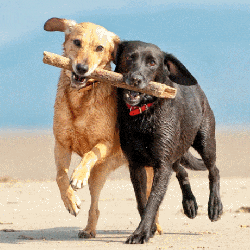
The results look fantastic, and there’s an interactive demo available where you can play with the different ways SAM works. One can pick out objects by pointing and clicking on an image, or images can be automatically segmented. It’s frankly very impressive to see SAM make masking out the different objects in an image look so effortless. What makes this possible is machine learning, and part of that is the fact that the model behind the system has been trained on a huge dataset of high-quality images and masks, making it very effective at what it does.
Once an image is segmented, those masks can be used to interface with other systems like object detection (which identifies and labels what an object is) and other computer vision applications. Such system work more robustly if they already know where to look, after all. This blog post from Meta AI goes into some additional detail about what’s possible with SAM, and fuller details are in the research paper.
Systems like this rely on quality datasets. Of course, nothing beats a great collection of real-world data but we’ve also seen that it’s possible to machine-generate data that never actually existed, and get useful results.
I’ll just leave this here. A treat for your M.2 socket https://hailo.ai/products/hailo-8-m2-module/
Similarly Googles Coral EdgeTPUs come with M.2, USB and Mini PCIe boards.
Mouser has the Mini PCIe version fully stocked (about £25 each).
It also has a semantic segment model available
https://coral.ai/models/semantic-segmentation/
It can only work with tflite models however. I don’t know about the accelerator you sent, but I was hoping for access to the matrix multiplication accelerator (Mac systolic array).
If this were applied to video and the frames stacked, to generate a simulation of the environment, it would be great for planning actions in robotics. Certainly closer to the way humans accomplish the task. Although I do wonder how portable the computing power to accomplish that amount of processing would be with today’s technology.
I know huge neural networks are the new hotness but it would be nice if someone wrote some code that would actually translate these highly functional NNs into actual logic that is then turned into machine code. It’s not impossible, it’s just a very difficult problem… which suggests that a neural network could be used to develop it.
c.f. whisper.cpp, llama.cpp
They already exist as code, but, your talking about turning it into if/else conditional logic such that you would be able to interpret it as a human. This is fundamentally impossible due to the way NNs work and the job they are doing, such that even tiring it into conditional logic, it’d be impossible for a human to read and understand it.
General computer vision systems are basically impossible to write as if/else conditional logic, because you might say; if(hasWings && hasFeathers && hasBeak) then return “bird”;
But writing code that can accurately determines if the picture contains feathers is nigh impossible, and you’d have to have code for every object and type of object the NN can identify.
If you want explainable NNs, what you need to do is run code that identifies which filters in which layers contribute to which results and manually research and tag them so that you can figure out which filter(s) contribute to Feathers, Beaks, Wings, Feet, etc. There already exist tools that do this, though, it is an evolving topic that is very labor intensive.
You should be able to find a starting point if you google “explainable AI Deep Learning”
This work, if successful, will be the pinnacle of work required to Reverse Engineer the human brain however – at a later time.