The current COVID-19 pandemic is rife with problems that hackers have attacked with gusto. From 3D printed face shields and homebrew face masks to replacements for full-fledged mechanical ventilators, the outpouring of ideas has been inspirational and heartwarming. At the same time there have been many efforts in a different area: research aimed at fighting the virus itself.
Getting to the root of the problem seems to have the most potential for ending this pandemic and getting ahead of future ones, and that’s the “know your enemy” problem that the distributed computing effort known as Folding@Home aims to address. Millions of people have signed up to donate cycles from spare PCs and GPUs, and in the process have created the largest supercomputer in history.
But what exactly are all these exaFLOPS being used for? Why is protein folding something to direct so much computational might toward? What’s the biochemistry behind this, and why do proteins need to fold in the first place? Here’s a brief look at protein folding: what it is, how it happens, and why it’s important.
First Things First: What Do Proteins Do?
Proteins are crucial to life. They provide not only the structural elements of the cell, but also serve as the enzymes that catalyze just about every biochemical reaction. Proteins, whether structural or enzymatic, are long chains of amino acids that are linked end-to-end in a specific sequence. The functions of proteins are determined by which amino acids are present at various locations on and in the protein. If a protein needs to bind to a positively charged molecule, for example, the binding site might be full of negatively charged amino acids.
To understand how proteins achieve the structure that defines their function, a quick review of the basics of molecular biology and the flow of information in the cell is in order.
The production, or expression, of a protein begins with the process of transcription. During transcription, the double-stranded DNA that holds the genetic information in a cell is partially unwound, exposing the nitrogenous bases of the DNA to an enzyme called RNA polymerase, often referred to as RNAPol. RNAPol’s job is to make an RNA copy, or transcript, of the gene. This copy of the gene, called messenger RNA or mRNA, is a single-stranded molecule that is perfect for directing the protein manufacturing machinery of the cell, the ribosomes, in a process called translation.
Ribosomes act like a jig, taking the mRNA template and matching it up to other small bits of RNA called transfer RNA, or tRNA. Each tRNA has two main active areas — one that has a three-base section called an anticodon that matches up with complementary codons on the mRNA, and a region for binding an amino acid that’s specific for that codon. During translation, tRNA molecules randomly try to bind to the mRNA in the ribosome using their anticodon. When a match is made, the tRNA molecule attaches its amino acid to the previous amino acid, forming another link in the chain of amino acids coded for by the mRNA.
This sequence of amino acids is the first tier of structural hierarchy in a protein, and is referred to as the protein’s primary structure. The entire three-dimensional structure of the protein, and indeed its function, comes directly from the primary structure through the different properties of each of those amino acids and how they interact with each other. If it weren’t for these chemical properties and interactions between amino acids, polypeptides would just remain linear sequences with no three-dimensional structure. We see this all the time in cooking, which is the heat-induced denaturation of the three-dimensional structure of proteins.
Long-Distance Connections Between Parts of Proteins
The level of structure beyond the primary structure is cleverly called the secondary structure, and includes fairly short-range hydrogen bonds between amino acids. These stabilizing interactions form two main motifs: the alpha-helix and the beta-pleated sheet. The alpha-helix forms a tightly coiled polypeptide region, while the beta-sheet is a flat, broad area. Both motifs have structural properties as well as functional properties, depending on the characteristics of the amino acids within them. For example, if an alpha-helix has primarily hydrophilic amino acids within it, like arginine and lysine, it’s likely to be involved in aqueous reactions.
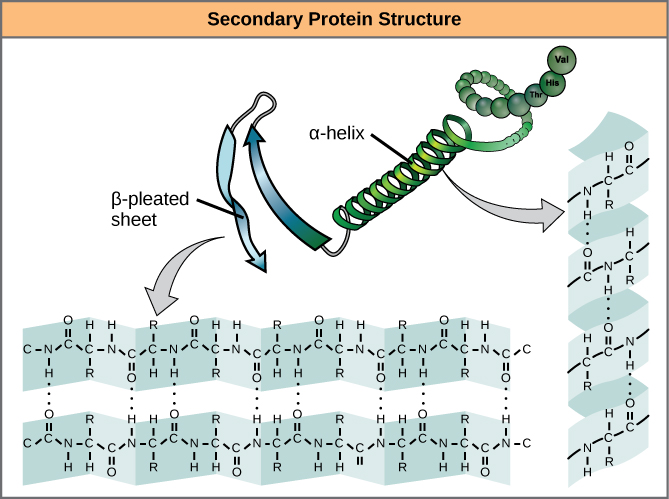
Proteins combine these two motifs, as well as variations on their themes, to form the next level of structure, the tertiary structure. Unlike the simple motifs of the secondary structure, tertiary structure tends to be driven more by hydrophobicity. Most proteins tend to have highly hydrophobic amino acids, like alanine and methionine, at their core, where water is excluded due to the “greasy” nature of the residues. These structures will often show up in transmembrane proteins, which are embedded in the lipid bilayer membrane surrounding cells. The hydrophobic domains on the protein are thermodynamically stable inside the fatty interior of the membrane, while the hydrophilic regions of the protein are exposed to the aqueous environment on either side of the membrane.
Tertiary structures also tend to be stabilized by long-distance bonds between amino acids. The classic example of this is the disulfide bridge, which often occurs between two cysteine residues. If you’ve ever been to a hair salon and smelled the slight rotten-egg stink of someone getting a perm, you’re witnessing the partial denaturation of the tertiary structure of keratin in hair by the reduction of disulfide bonds using sulfur-containing thiol compounds.
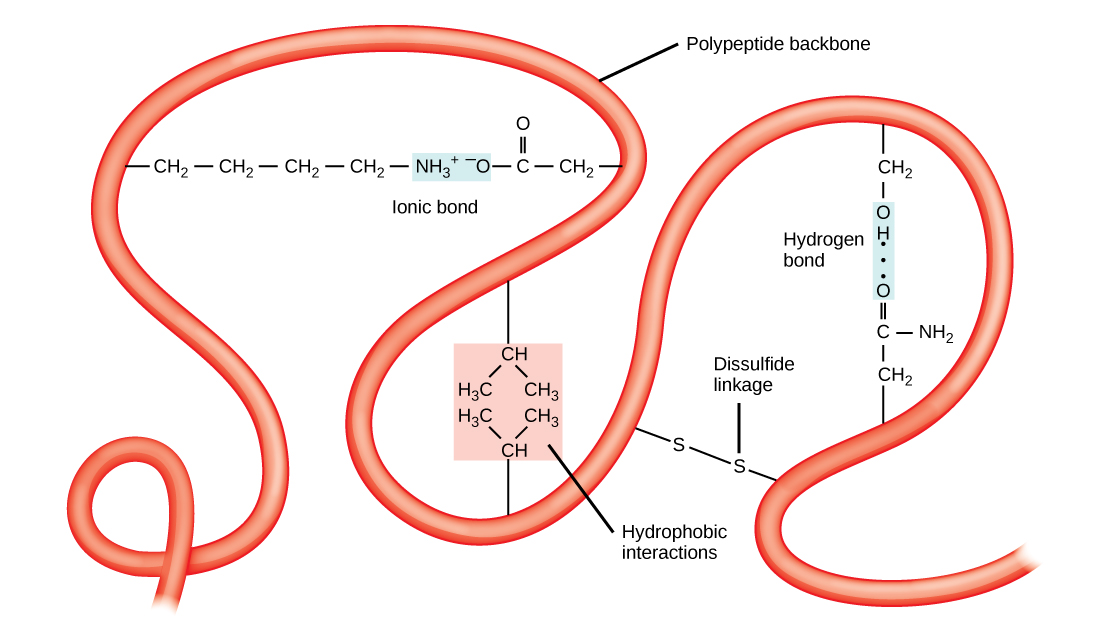
Disulfide bridges can occur between cysteine residues in the same chain of polypeptides, or between cysteines locate in completed different chains. Interactions between different polypeptide chains are the fourth level of protein structure, the quaternary structure. The hemoglobin in your blood is a perfect example of quaternary structure. Each hemoglobin molecule is formed by four identical globin protein subunits, each of which is held in a specific conformation by disulfide bridges within the polypeptide as well as bonding with the iron-containing heme molecule. All four globin subunits are bound together by intermolecular disulfide bridges, and the entire molecule acts as one to bind up to four oxygen molecules at once, and to release them when needed.
Modeling Structures In a Search for Solutions to the Illness
Polypeptide chains begin folding into their final shape during translation, as the growing chain is extruded from the ribosome, similar to the way a piece of straightened memory wire can snap into a complex shape when heated. But as is always the case with biology, there’s much more to the story.
In many cells, there is extensive editing of the transcribed genes that occurs before translation, which alters the primary structure vastly compared to the raw base sequence of the gene. The translational machinery also often enlists the help of molecular chaperones, proteins that temporarily bind to the nascent polypeptide chain to prevent it from taking an intermediate structure that would prevent it from taking its final shape.
All this is to say that predicting the final shape of a protein from the primary structure is not trivial. For decades, the only way to explore protein structure was with physical methods like X-ray crystallography. It wasn’t until the late 1960s that biophysical chemists started building computational models for protein folding, focused mainly on modeling the secondary structure of a protein. These methods and their descendants take a vast amount of input data in addition to the primary structure sequence, such as tables of bond angles between amino acids, lists of hydrophobicity, charge states, and even conservation of structure and function over evolutionary timescales to make a best guess at what a protein is going to look like.
Current computational methods for secondary structure prediction, like those running on Folding@Home’s network right now, run at about 80% accuracy, which is pretty good considering the complexity of the task. The data generated by the folding prediction models for proteins like the SARS-CoV-2 spike protein will be coupled with physical study data to come up with a firm structure for the protein, and perhaps give us insights into how the virus binds to the human angiotension converting enzyme-2 (ACE-2) receptors that line the respiratory tract, which is its path into the body. If we can figure out the structure, we might be able to find drugs to block binding and prevent infection.
Protein folding research is central to our understanding of so many diseases and infections that even once we figure out a way to beat COVID-19, the Folding@Home network, which as seen such explosive growth over the past month, will not go idle for long. The network is a research tool well-suited to exploring protein models central to dozens of diseases that are related to misfolded proteins, such as Alzheimer’s and variant Cruetzfeldt-Jakob disease, often incorrectly called mad-cow disease. And when the next virus inevitably comes along, all that horsepower, and all the experience being gained in managing it, will be ready to go again.
I gather that, in addition to actual protein folding simulations, the folding@home system is also being used to do other covid-19 related computations (like in-silico docking of potential small-molecule inhibitors of the main covid protease etc.):
https://discuss.postera.ai/t/docked-covid-moonshot-compounds/576
I wonder if someone here has enough insight to tell us if quantum computers would actualy help the covid-19 research in some meaningful way providing practical benefits…
Yes, around about the time we get hydrogen powered flying cars and too cheap to meter fusion power.
The number of qubits in these current lab experiments are highly limited and they do toy proof of concept problems. IDK really if it’s even reached the equivalent of the 4004 CPU yet, it’s more like room sized UNIVACs (considering all support equipment)
https://physics.aps.org/articles/v12/112
This article answers your question.
Trying to analogise, bearing in mind all analogies are limited…
So we’ve got a cell which has a nano 3D printer, the SBC running it has a ROM with it’s base code to reproduce itself, however, the bus lines are all exposed, spread all the heck everywhere. Then we’ve got this scheming little bugger the virus, which has a malicious USB stick, with just the right spread of contacts to insert fake code to the printers SBC makig it produce copies of the virus, not it’s own DNA. Thinking 2 dimensionally, this might be like a test jig that hits vulnerable points on the SBC that allows service/reflash or in this case pwnage. However, this is all really tiny and in 3 dimensions, so figuring out which bus lines the sploit uses is extremely complex. When we know that, we can do the equivalent of filling the USB ports with epoxy or otherwise disable the USB stick of the virus before it gets plugged in. Added to that, the virus is disguising the interface, so James Bond style it folds up into an everyday object to get it through customs, and will only assemble it to the right shape to interface when it’s getting ready to do so. So you look at the virus holding it, looks like a cassette tape, you KNOW it’s a decepticon, but what shape does it transform into to make that interface? So when you see that and know there’s a billion points the hinges could be, a billion points where the contacts could be, and a billion points in space where they could be hinged out to, you get why it takes a lot of FLOPs to figure it all out.
Serious question here. So if Folding@Home is helping us to come up with an educated guess at what the secondary structure is; How do we then go about confirming our findings?
By comparing to the real structure:
https://www.nih.gov/news-events/nih-research-matters/novel-coronavirus-structure-reveals-targets-vaccines-treatments
This was solved by cryo-EM, which has taken the world of structural biology by storm in the last few years and is increasingly (if not already) becoming a serious contender to protein X-ray crystallography.
I think I misunderstood initially. So you’re saying we can can view the shape of the actual structure.. but what we’re trying to do is determine the sequence of amino acids that make up the proteins that dictate the physical structure of the virus? We then assume we’ve discovered the proper sequence/composition when the simulated structure matches the observed structure?
Usually determining the amino acid sequence is more straightforward than getting the 3D structure (you can do it chemically without having a crystalline sample and access to x-ray crystallography, although this might take a while if you don’t have automation and might need a lot of sample). If you have a crystal of protein and the right kit, you can get the 3D structure which also gives you the amino acid sequence automatically. This used to be very difficult, but it has become a lot more ‘routine’ in the last couple of decades.
@lars-mander Now I’m completely lost. What is the point of folding@Home then? What is the purpse?
A big part of what folding@home is doing is not just about the lowest energy structure of the protein but about how the protein moves dynamically (which you cannot easily get experimentally). There are now many ‘actual’ 3D structures of several of the SARS2-nCOV proteins, including the main MPRO protease, including many with real molecules bound to the active site. I think these were mainly obtained using x-ray crystallography (the Diamond light source in the UK has obtained many of these):
https://www.diamond.ac.uk/covid-19/for-scientists/Main-protease-structure-and-XChem/Downloads.html
(they have an interactive 3D viewer or you can just download the structures to view in pymol or whatever).
These small molecules are probably not suitable as actual drugs, but are a good starting point for further design:
https://covid.postera.ai/covid
The static 3D structure of the protein, with or without other molecules bound to it, gives a lot of really useful information, especially about the active site (where the catalytic reaction takes place), but proteins are not static. They can often change shape when molecules bind to them, including at sites other than the active site. When this happens, the active site can also change shape. This is called allosteric binding and several drugs work in this way:
https://en.wikipedia.org/wiki/Allosteric_modulator
https://en.wikipedia.org/wiki/Allosteric_regulation
So, knowing how the protein moves ‘mechanically’ opens up new ‘druggable’ sites.
I really appreciate you taking the time to thoroughly explain this to me.
Please don’t forget Rosetta@Home. https://boinc.bakerlab.org/rosetta/
YADCE (Yet Another Distributed Computing Effort)
Isn’t it more useful to jump on the ones others use, as to not crunch the same numbers twice?
The two projects are complimentary, not opposing. I think I heard they even use the findings from one to fuel the research for the other. Many people run them at the same time on the same computer, even.
Impressively the ‘spike’ protein structure has already been solved experimentally.
https://www.nih.gov/news-events/nih-research-matters/novel-coronavirus-structure-reveals-targets-vaccines-treatments
It will be interesting to see how this “real” structure compares to the in silico prediction from Folding@Home. We should maintain some healthy skepticism regarding these protein folding programs. The accuracy of these computational simulations, though intensive, depend fundamentally on the quality of the modeling of the intramolecular interactions and the relative weighting of the various types of interactions (the “scoring” function) – inevitably how the trade-off for speed vs accuracy can match reality. We need better scoring functions, better modeling of reality – throwing more computers at the job isn’t enough by itself. Personally, I have wondered whether this might be something machine learning can help with, rather than this brute force simulation approach. We need a bright spark to download protein structures from the PDB (Protein DataBase), analyse the amino acid sequence, and get to work with their machine learn algorithms of choice to predict the 3D structure from the sequences…
Just my tuppence worth!
Isn’t the problem with that one of requiring effective multitudes of folding@home clusters while the algorithm practises with the training data?
I’m not completely sure what your question refers to (what is “that”?), but the folding@home algorithm will have been trained on at least *some* of the solved structures from the PDB. I’m guessing that 80% accuracy figure quoted refers to folding@home prediction versus PDB structure reality. There’s probably a paper to go and look up if we are really interested!
We’re working on it! https://github.com/jonathanking/protein-transformer
One of the reasons this is so difficult is that all the interactions are entropically favorable. The virus proteins fold up into the right shape automatically, because that’s their thermodynamically most favorable shape. The S protein that forms the spikes we see in drawings of the virus sticks out and contacts the cell surface, and because of the lower pH adjacent to the cell surface, the S protein changes its shape, which presses the virus membrane into contact with the cell membrane and causes them to fuse. Once inside the cell, the RNA looks just like existing cellular RNA. It’s difficult to find places that can be blocked.
In addition to that, it’s such a noisy, high amplification system that even if you make a version of the virus that has changed RNA so it won’t be as pathogenic, but will still stimulate an immune response, it’s pretty common for the virus to have replication errors that re-create the original virus, because that’s what evolution does. The best-propagating version gets amplified the most.
“One of the reasons this is so difficult is that all the interactions are entropically favorable. ”
Thermodynamically favorable, yes… all interactions are entropically favourable… no, or if they are (and that’s not demonstrated), it is not really relevant! :) All proteins fold up into their thermodynamically most favorable shape because, well, thermodynamics. The S protein is nothing different in that regard. The spike protein is thought to interact with the human angiotension converting enzyme-2 (ACE-2) receptors on the cell surface, not to do with “local lower pH”. This interaction with ACE-2 is a tentative drug target, as is the methyltransferase nsp10/nsp16, which modifies the RNA of the virus to make it more like human RNA. Luckily there are many smart people working on it to think about lots of different ideas for how we might tackle this disease :)
Folding hasn’t produced any medically usable therapies in over ten years of operation (although it has produced several “promising” ones). Long term it’s probably useful, but it’s not going to be the magic bullet that cures corona any time soon, certainly not as quick as we need.
Fair point, but the folding@home system is also doing a lot of other non-folding computations, including simulated docking of small molecules. Those that look promising will be synthesized and tested against real protein (many are already being synthesized now). These may also include existing drug compounds (although I don’t know for sure about that).
https://foldingathome.org/2020/03/30/covid-19-free-energy-calculations/
So, there are lots of reasons to donate cpu time, it’s not just about folding/dynamics.
That image is golden XD
perhaps some more advances would be made possible applying openCV to it, as the foldit project is doing, geez I wish I knew more python or some other language, on the other side, releasing skynet to fight coronavirus might not be a good idea after all.
anyway, thanks for the clear explanation in the article, always wanted to know.
Just wanted to point out Team Hack-a-Day on Folding@Home is now in the top 50 teams as of 20200416, we have gained a lot of momentum with the interest in COVID-19; let’s keep it up.