Anyone who’s ever tried their hand at reverse engineering a piece of hardware has wished there was some kind of magic wand you could tap on a PCB to understand what its doing and why. We imagine that’s what put security researcher [Mark C] on the path to developing CurrentSense-TinyML, a fascinating proof of concept that uses machine learning and sensitive current measurements to try and determine what a microcontroller is up to.
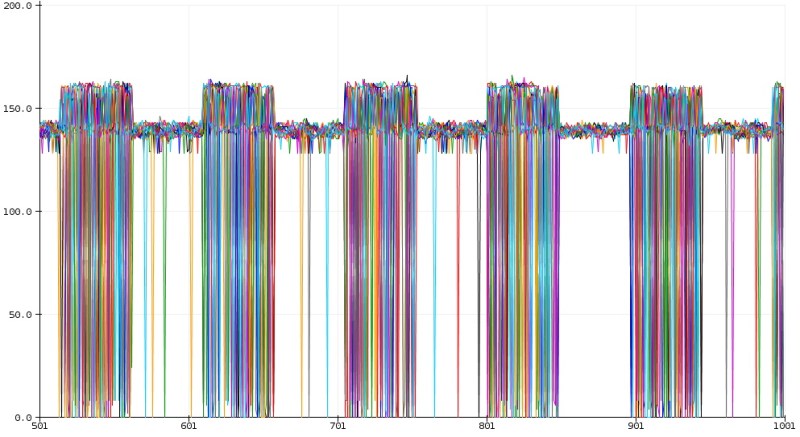
The idea is simple enough: just place a INA219 current sensor between the power supply and the microcontroller under observation, and record the resulting measurements as it goes about its business. Of course in this case, [Mark] knew what the target Arduino Nano was doing because he wrote the code that blinks its onboard LED.
This allowed him to create training data for TensorFlow, which was ultimately optimized into a model that could fit onto the Arduino Nano 33 BLE Sense which stands in for our magic wand. The end result is that the model can accurately predict when the Nano has fired up its LED based on the amount of power it’s using. [Mark] has done a fantastic job of documenting the whole process, which also doubles as a great intro for putting machine learning to work on a microcontroller.
Now we already know what you’re thinking: obviously the current would go up when the LED was lit, so the machine learning aspect is completely unnecessary. That may be true in this limited context, but remember, this is just a proof of concept to base further work on. In the future, with more training data, this technique could potentially be used to identify a whole range of nuanced activities. You’d be able to see when the MCU was sitting idle, when it was writing to flash, or when it was reading from sensors. In fact, with a good enough model, it might even be possible to identify the individual sensors that are being polled.
These are early days, but we’re very interested in seeing where this research goes. It might not be magic, but if analyzing the current draw of a coffee maker can tell you how much everyone in the office is drinking, then maybe it can help us figure out what all these unlabeled ICs are doing.