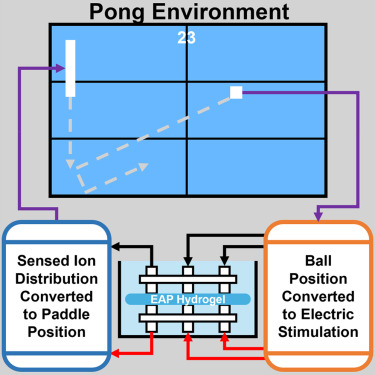
Of the many big, unanswered questions in this Universe, the ones pertaining to the functioning of biological neural networks are probably among the most intriguing. From the lowliest neurally gifted creatures to us brainy mammals, neural networks allow us to learn, to predict and adapt to our environments, and sometimes even stand still and wonder puzzlingly how all of this even works. Such puzzling has led to a number of theories, with a team of researchers recently investigating one such theory, as published in Cell. The focus here was that of Bayesian approaches to brain function, specifically the free energy principle, which postulates that neural networks as inference engines seek to minimize the difference between inputs (i.e. the model of the world as perceived) and its internal model.
This is where Electro Active Polymer (EAP) hydrogel comes into play, as it features free ions that can migrate through the hydrogel in response to inputs. In the experiment, these inputs are related to the ball position in the game of Pong. Much like experiments involving biological neurons, the hydrogel is stimulated via electrodes (in a 2 x 3 grid, matching the 2 by 3 grid of the game world), with other electrodes serving as outputs. The idea is that over time the hydrogel will ‘learn’ to optimize the outputs through ion migration, so that it ‘plays’ the game better, which should be reflected in the scores (i.e. the rally length).
Based on the results some improvement in rally length can be observed, which the researchers present as statistically significant. This would imply that the hydrogel displays active inference and memory. Additional tests with incorrect inputs resulted in a marked decrease in performance. This raises many questions about whether this truly displays emergent memory, and whether this validates the free energy principle as a Bayesian approach to understanding biological neural networks.
To the average Star Trek enthusiast the concept of hydrogels, plasmas, etc. displaying the inklings of intelligent life would probably seem familiar, and for good reason. At this point, we do not have a complete understanding of the operation of the many billions of neurons in our own brains. Doing a bit of prodding and poking at some hydrogel and similar substances in a dish might be just the kind of thing we need to get some fundamental answers.