If you’ve looked into GPU-accelerated machine learning projects, you’re certainly familiar with NVIDIA’s CUDA architecture. It also follows that you’ve checked the prices online, and know how expensive it can be to get a high-performance video card that supports this particular brand of parallel programming.
But what if you could run machine learning tasks on a GPU using nothing more exotic than OpenGL? That’s what [lnstadrum] has been working on for some time now, as it would allow devices as meager as the original Raspberry Pi Zero to run tasks like image classification far faster than they could using their CPU alone. The trick is to break down your computational task into something that can be performed using OpenGL shaders, which are generally meant to push video game graphics.
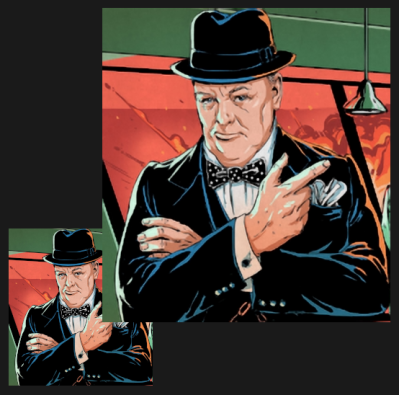
[lnstadrum] explains that OpenGL releases from the last decade or so actually include so-called compute shaders specifically for running arbitrary code. But unfortunately that’s not an option on boards like the Pi Zero, which only meets the OpenGL for Embedded Systems (GLES) 2.0 standard from 2007.
Constructing the neural net in such a way that it would be compatible with these more constrained platforms was much more difficult, but the end result has far more interesting applications to show for it. During tests, both the Raspberry Pi Zero and several older Android smartphones were able to run a pre-trained image classification model at a respectable rate.
This isn’t just some thought experiment, [lnstadrum] has released an image processing framework called Beatmup using these concepts that you can play around with right now. The C++ library has Java and Python bindings, and according to the documentation, should run on pretty much anything. Included in the framework is a simple tool called X2
which can perform AI image upscaling on everything from your laptop’s integrated video card to the Raspberry Pi; making it a great way to check out this fascinating application of machine learning.
Truth be told, we’re a bit behind the ball on this one, as Beatmup made its first public release back in April of this year. It might have flown under the radar until now, but we think there’s a lot of potential for this project, and hope to see more of it once word gets out about the impressive results it can wring out of even the lowliest hardware.
[Thanks to Ishan for the tip.]