DreamWire is a system for generating multi-view wire art using machine learning techniques to help generate the patterns required.
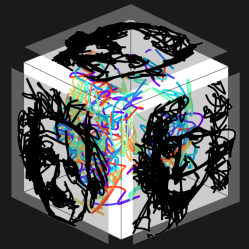
What’s wire art? It’s a three-dimensional twisted mass of lines which, when viewed from a certain perspective, yields an image. Multi-view wire art produces different images from the same mass depending on the viewing angle, and as one can imagine, such things get very complex, very quickly.
A recently-released paper explains how the system works, explaining the role generative AI plays in being uniquely suited to create meaningful intersections between multiple inputs. There’s also a video (embedded just under the page break) that showcases many of the results researchers obtained.
The GitHub repository for the project doesn’t have much in it yet, but it’s a good place to keep an eye on if you’re interested in what comes next.
We’ve seen generative AI applied in a similarly novel way to help create visual anagrams, or 2D patterns that can be interpreted differently based on a variety of orientations and permutations. These sorts of systems still need to be guided by a human, but having machine learning do the heavy lifting allows just about anybody to explore their creativity.